People. Process. Tooling. This is the trifecta; the ‘secret sauce’ that critically defines and underpins any successful digital transformation project, at any scale. This isn’t to say you must have all three in equal parts to be successful. Rather you have to have an understanding of how all three fit into the new world that you’re building for your organization to thrive, grow and adapt. Businesses are constantly changing and the demand for, and ability to provide high-quality, trusted data are at the heart of most existing and emerging initiatives like AI & MLOps, Data Mesh & Fabric or Real Time Data analysis.
While data catalogs have emerged as a collaborative and well-governed method for connecting people to data to help support these initiatives, driving their adoption and growth within organizations can be surprisingly challenging. Many attempts fall short of initial expectations, leaving users frustrated and questioning the platform’s true value. What key ingredients could be missing for truly successful data catalog implementations?
Data context is missing
When relying on the data sources, connections and the purely technical (the ‘Tooling’), or the ownership and roles within the catalog (the ‘People’), we realize that we’re missing some of the most crucial elements of a data intelligence approach, which creates pitfalls in understanding the “why” behind the data. Without the context, we are unable to answer questions like:
- Why certain data products were made available in the first place
- Why decisions were made around the usage of, and how people should get access to, data
- Why some teams are using different reports or sets of data (potentially for seemingly similar purposes)
Without the why (and by extension the how) we use a data catalog to create a central resource that holds a lot of our collective knowledge and can be useful in solving very technical challenges, but which lacks a lot of our collective understanding. The catalog acts as a window into our data estate as it is today; what the fundamental structure of the schemas, tables and columns is, the lineage between tables and views and which BI reports that data flows into. What it doesn’t show us is:
- Why lineage isn’t available potentially due to manual process steps
- Any of the ad-hoc tasks we carry out infrequently e.g. at year end
- If and how the data was signed off by compliance for use in our latest predictive models
These are significant gaps in both our understanding of how we are using data, as well as how we are supposed to use data. Although a data catalog should help us break down data silos, the above approach leaves us with more questions and reinforces the idea that data is a hotly guarded secret.
Level up to a process-aware catalog
When beginning to roll out a data intelligence platform, it is always important to not “boil the ocean” and to follow a use case based approach — but each of these use cases around data may have existing processes.
Documenting existing processes in the catalog itself by creating a process register, alongside the metadata, business terms, policies and quality markers for data, creates a complete view of the lifecycle of data, without relying only on what can be automatically harvested. This is a “process-aware” catalog.
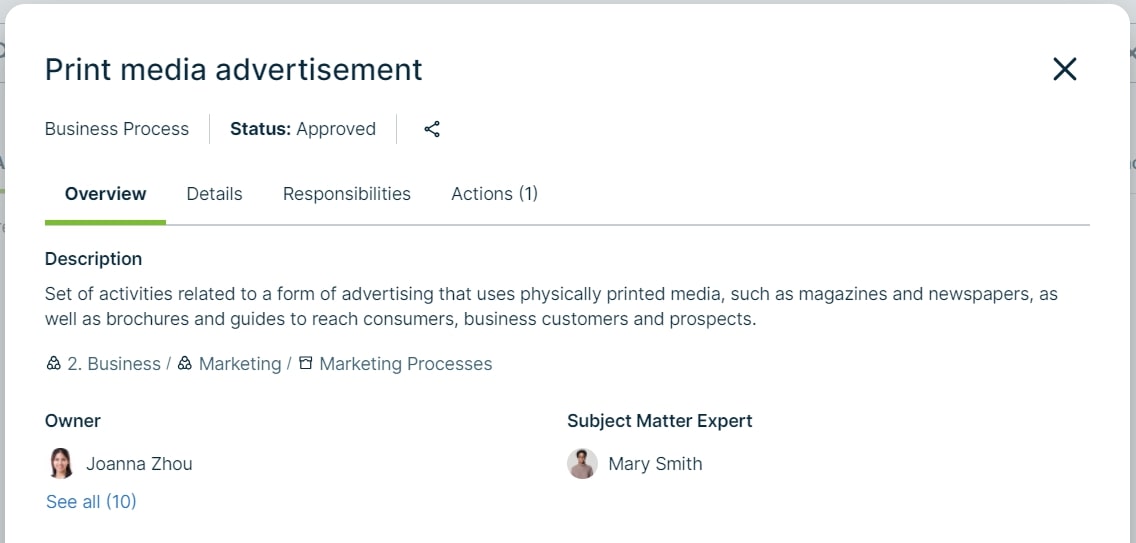
Figure 1: An example of a documented, mostly manual but still data consuming, business process for “Print media advertisement” in Collibra with a description and ownership, which helps people understand the necessary commercial purpose of this process
With each set of metadata that is harvested, we can invest the time to document and govern the critical processes carried out by the teams and stewards who look after and consume that data. By speaking to stakeholders and identifying key process milestones, building out a view of these processes and mapping what data sources are involved as well as how critical they are, we can build up an overall idea of the data process health of the organization, and what our first priorities for process improvement could be.
Once documented in the catalog, the process itself can then be attached to give wider context not only to the metadata itself, but also show:
- The lines of business who own and govern the process
- The policies or assessments internally that govern the process and it’s periodic review
- Any risk that may be associated with that process and the purpose or justification for it
Here is one example of what a mapping may look like – the purpose of this diagram is to understand which line of business’ process we may impact by making schematic changes to the underlying physical data:
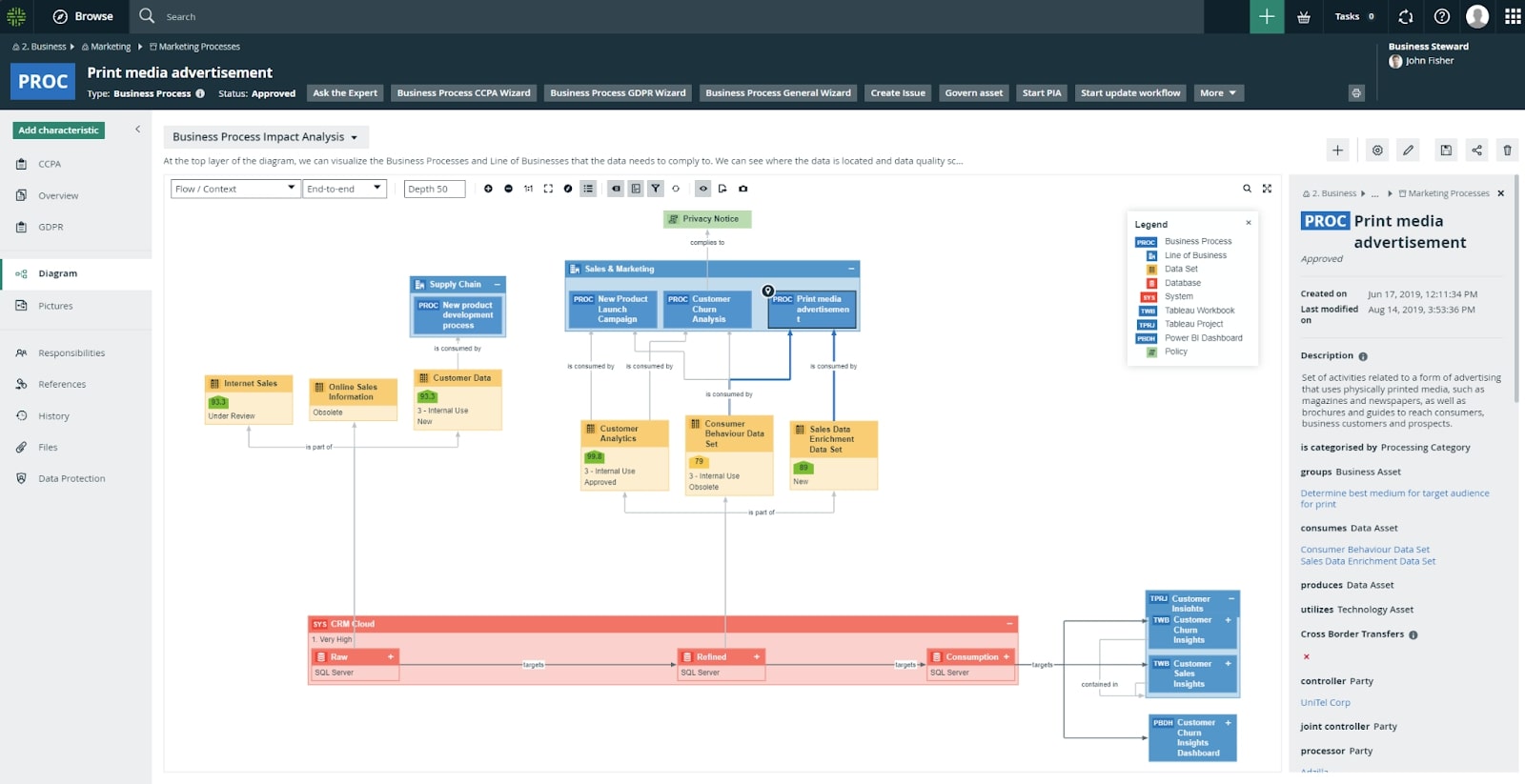
Figure 2: An example of a Business Process impact mapping diagram in Collibra showing the lineage from the “Print Media Advertisement” process and how you can identify which reports, processes and teams you may impact by making structural or data changes
With this approach we can easily tackle the significant gaps we identified. In our examples from earlier we can easily map data from one system to another via the process, to represent the missing manual step(s). We can even solve the compliance issue of understanding how data was signed off by documenting the compliance process so everyone in the organization knows how to properly request data for AI models, for example. If we want to go a step further, we can even automate this request process using governance workflows within the catalog itself.
No more “data mysteries”
By including well-documented, governed business processes alongside your technical metadata, you have the perfect starting point from which to drive value. Combining comprehensive technical metadata and lineage with business lineage enables us to understand our most mission critical processes, how and why data is used, and crucially how the rest of the organization works. This ultimately helps us:
- Enhance data transparency and context: New joiners, internal movers, legal and compliance… The list is extensive for the roles at the organization who are able to benefit from better understanding processes from start to finish which breaks down data silos, minimizing the reliance on old, out-of-date documentation and helping reduce risk of manual errors.
- Streamline data collaboration and automation: The right people are able to find and trust the right data for their needs much faster. Not only are they able to find where the data is, how it is transformed and if it’s high quality, but they understand where issues might be introduced and who else within the organization they may need to work together with to ensure it is used correctly.
- Boost data governance, compliance and organizational efficiency: Understanding data usage helps everyone within the organization understand from the very beginning how data is used, meaning we can take the right steps early on to safeguard it, assess and evaluate it regularly, approve access to it and put the right guardrails in place when processing it (like conducting a Data Processing Impact Assessment, or DPIA).
Including your business processes alongside the metadata can be transformational both for technical and business stakeholders, and give you the level of explainability that can help drive people to your catalog for quick, accurate answers about data or processes anywhere in the organization.
Interested in finding out about how you can document your processes and metadata together in Collibra? Get in touch today.