Data engineers play a critical role in powering business analytics, ML, and data products. Their focus is on providing data product owners, data analysts, data scientists and decision-makers with reliable and high-quality data.
With high data volumes and diverse sources, it is likely to have data that is duplicate, incomplete, inconsistent, or inaccurate. If not addressed in time, these issues can propagate across the entire data ecosystem of the organization. Data engineers constantly monitor the health of data pipelines to catch such errors before they can hurt.
Data observability for data health
Traditional data quality tools rely on finding and fixing errors with manual or partially automated rules. However, these tools cannot cope with the high volumes of rapidly streaming data. The three concerns data engineers have with these tools are:
- No real-time visibility into data health.
- More applications are affected before detecting and fixing the errors.
- High cost of writing and maintaining data quality rules.
A comprehensive approach to address these concerns is Data Observability. It is a set of tools to track data health as data moves through the enterprise systems. Data observability continuously monitors the five pillars to enable a wider view of data, with context, business impact, lineage, performance, and quality. It provides the ability to monitor data continuously, detect issues proactively, and help resolve them before they hurt.
Data engineers are familiar with system observability, where external outputs are used to track and improve the system health. In the same way, data observability helps track and improve data health.
Collibra Data Quality & Observability proactively surfaces quality issues in real time with auto-discovered and adaptive data quality rules. Making reliable data available to drive trusted business decisions, it empowers to:
- Get real-time, end-to-end visibility into the health of data.
- Discover real-time breaking trends with ML-generated, adaptive, and explainable rules and prevent bad data from making its way downstream.
- Reduce data downtime with proactive anomaly detection. Scan large and diverse databases, files, and streaming data to get 90%+ coverage at scale.
- Carry out row, column, conformity, and value checks between source and target data storage.
- Deliver data pipeline observability in just one sprint.
- Leverage low-code, API-based integration to get quickly running with minimal pipeline changes.
Gartner Research notes that data observability has now become essential to support as well as augment existing and modern data management architectures. A quick comparison shows how data observability augments the current data quality efforts.
Traditional data quality tools | How data observability tools augment data quality |
Monitor datasets. | Monitor datasets (data at rest) as well as data pipelines (data in motion). |
The approach is finding ‘known’ issues with data | The approach is in understanding data with context and detecting ‘unknown’ issues with ML-generated adaptive rules. |
Help data stewards and business analysts measure and maintain data quality. | Enable data engineers and DataOps engineers to deliver reliable and trusted data across the enterprise. |
Do not support root cause investigation. | Enable root cause investigation through lineage, time series analysis, and cross-metric anomaly detection. |
The focus is on downstream use cases of trusted reporting and compliance. | The focus is on upstream use cases of anomaly detection, pipeline monitoring, and data integration. |
Much more than monitoring, data observability identifies changes in data or patterns to detect unknown issues early. It alerts to enable analysis and delivers reliable data across the enterprise.
Key use cases of data observability
The key use cases for leveraging data observability include:
- Accelerated cloud data migration with complete data integrity validation between source storage and target data lake.
- Efficient management of data lake health with proactive monitoring to identify missing or incomplete data.
- Cost optimization by improving operational efficiency of data pipelines and streamlining DataOps.
- Faster time to analytics as a result of reduced data downtime and healthier data pipelines.
- Rapid AI adoption with robust data pipelines and continuous improvement in data health.
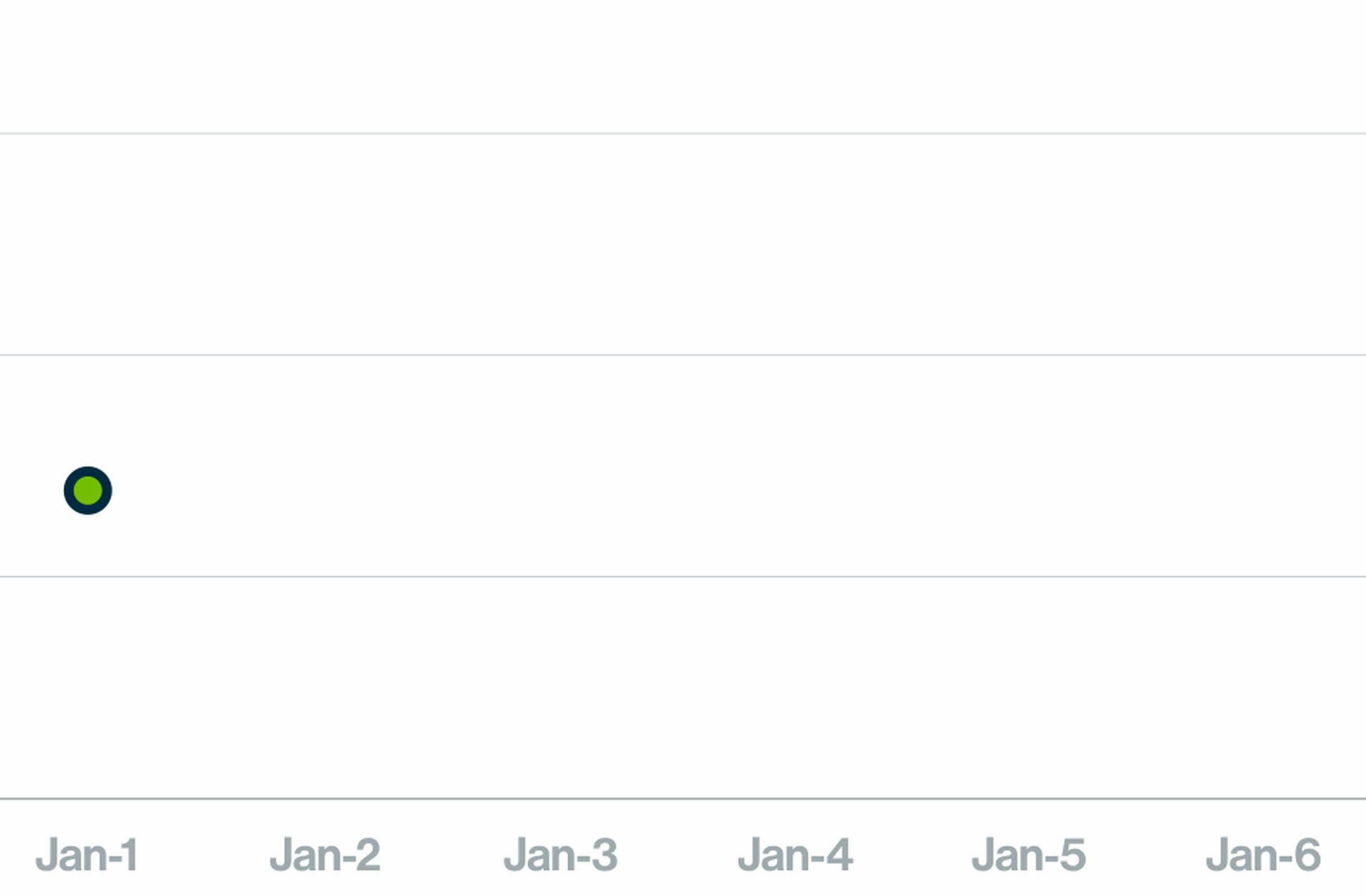
Data pipeline observability for operational health monitoring: Avoid data downtime with proactive monitoring and anomaly detection
How data observability helps data engineers
Data observability proactively monitors and detects issues early to deliver reliable and trusted data across the enterprise.
1. Improves data reliability
Data reliability is the ability to deliver high data health and availability throughout the data lifecycle. It is often more relevant than just data quality, as it indicates that trusted data is ready for use in applications and analytics.
Assuring data reliability for large volumes of diverse data is always challenging. Data observability can help there with real-time visibility into data stores and pipelines. Constant monitoring and proactive anomaly detection reduce the likelihood of poor-quality data. Finally, leveraging ML for adaptive rules significantly cuts down manual work and improves data reliability at scale.
2. Helps build healthier data pipelines
Data pipelines use a set of tools and processes to automate the movement and transformation of data from ingestion to consumption. They are now indispensable for powering complex analytics and data-driven decisions.
Building healthy pipelines begins with discovering data, understanding the context behind data, and then building trust in it. Data engineers spend a great deal of time and effort on these activities instead of focusing on innovative projects. Very large volumes of data with diverse sources and hybrid data storage add to their challenges.
Context is critical in understanding data and assessing its health. Data observability delivers the visibility and context required to diagnose data health issues. It uses continuous monitoring, automated quality checks, and proactive issue detection to help build healthier data pipelines.
3. Increases operational efficiency
Data observability takes a more comprehensive approach with context and performance of data, in addition to data quality. The use of ML helps determine issues much earlier and beyond the known metrics. These features ensure faster delivery of healthier data, taking the uncertainties out of using trusted data for enterprise operations.
It also ensures more efficient data migrations by automating data validation and reconciliation. Collibra automates critical data quality workflows for data producers and consumers to further increase operational efficiency.
4. Streamlines DataOps
DataOps bridges data producers and consumers. DataOps is a set of practices & technologies to build data products and operationalize data management to improve quality, speed, and collaboration and promote a culture of continuous improvement.
-Source: https://www.sanjmo.com/
Data observability empowers DataOps and data engineers to profile data in motion and identify failures. With lineage, it is possible to track data to the point of failure, perform root cause investigation, and fix issues at the source.
The ML-driven, adaptive, no-code approach to data quality simplifies rule management. Auto-generated rules help quickly create monitoring controls and instantly detect data issues. If custom rules are required, the templates help build reusable, shareable rules and streamline DataOps.
5. Powers FinOps
Cloud computing has undoubtedly reduced the burden of high Capex. But organizations still need to keep an eye on their Opex. FinOps is a framework and management practice that promotes shared responsibility for managing Opex across the organization’s cloud computing infrastructure. The objectives of FinOps are to establish best practices to optimize costs, deliver business value, and maintain financial accountability.
Data observability helps build trusted data pipelines to accelerate data-to-value. It efficiently tracks the health of data across the cloud infrastructure to minimize costs and maintain compliance with regulations. The ML-driven, autogenerated, adaptive rules of Collibra catch data errors early and keep the rework costs down.
In summary
Data observability offers a modern approach to reliable and trusted data with five pillars of context, business impact, lineage, performance, and quality. It actively leverages ML to manage data health in the face of increasing volume, diversity, and speed of data.
Forbes notes that data management complexity will continue to increase, which means continued and dedicated attention to data engineering is needed. Collibra enables data engineers to build healthier data pipelines, improve data reliability, and deliver trusted data products at scale.