As a former Chief Product Owner managing technology for a digital transformation, I found that our greatest success was not just throwing software at a problem, but holistically considering it in the context of people, process, and technology. Technology had the largest budget, but our Agile Center of Excellence drove the adoption (and at times enforcement) of technology.
It’s this successful model in Digital Transformations that likely drives concepts like data mesh. Data organizations are realizing that no amount of technology can solve for good programmatics around the people and process. Yes, the biggest budget allocation will be for technology with needs like data migration or a new privacy application, but the biggest focus will have to be people and process. I think data mesh is the conceptual way of tying technology to people and processes and enabling an organization to improve its data governance.
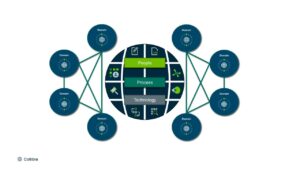
It’s easy to hear “data mesh” and think of only technology
Why an approach and not just a technology?
We should not forget the origins of service-mesh technology and its applicability to Data Mesh. Service mesh is an architectural concept in technology to help foster the speed of interaction between an application and its need to compute. Conceptually, it helps enable things like microservices and avoids the dreaded “monoliths.” These concepts correspond to tying an application closer to its data and thus an opportunity for Data Governance.
If a business sees itself as originating data, but then is also a consumer of data – they would want to have a say in the aspects of data governance. While they might leverage centralized metadata for compute, they would want to retain ownership without having to centralize the data itself. Perhaps the business could own their data without governing it from the CDO (avoiding a monolith). In comes data mesh, “A decentralized sociotechnical approach in managing and accessing analytical data at scale.”
Data mesh provides the ability to foster communications between the business – originators of the data – and the stewards/technologists who will help organize the data. Ultimately, the concept supports decentralization, helping maximize contribution from all. Data mesh is not just a technology but an architecture around people and processes. It will enable your originators to be a part of the data landscape and speed up the data-to-value equation.
Unfortunately mesh concepts are not an easy transition. Service mesh similarly required a change in the paradigm of software development. For example, Kubernetes is something that monolithic architectures still struggle to support. In order to better support the transition to mesh, we consider what may enable decentralized people to enact their own process around data and its governance. It’s key that enabling a decentralized program still requires aspects of centralization.
Consider a topic like “flattening communications.” With email, any group of people is only clicks away from generating a forum. To manage the possible chaos, we have select processes in place like regulating the email distro size. That example suggests that centralization will be key for certain aspects of the people, process, and technology model.
A CDO would then ideally want to have a consensus on processes to help enable the successful data mesh. In order to keep consistent governance, the office might more fully centralize technology to ensure consistent inputs. This resonates with a CTO who might enforce a standard for technical documentation with “everything is a ticket” – then leveraging ServiceNow as the system of record.
So then, how do we mesh our Data Observability?
To quote from a previous blog, Data Observability is now rapidly gaining momentum in DataOps, delivering a deep understanding of the data systems and full business context of data quality issues. These capabilities continuously monitor the five pillars, alerting DataOps before any data issues can edge in. In the coming years, data observability will be considered a critical competency of data-driven organizations.
As we see technology centralized for consistency but decentralized for participation, it’s important to have technology that allows for multiple contributors beyond a data steward or a data engineer. Data quality anomalies will be best understood by the upstream business domains. A successful data mesh then includes desegregated data observability, and Collibra Data Quality & Observability strives for the data mesh approach with the below value offerings:
- It provides insight in a statistical sense to avoid the complexity of technical output. One should not have to read SQL to understand the quality of data.
- It offers rule templating and discovery to minimize the data engineer efforts. One should not have to write SQL to enforce the quality of data.
- It still supports an engineering approach with full API integration. One should not have to use the UI in their data engineering use case.
To conclude, as our data world moves towards infrastructure built in concepts like data mesh, we see a need for data quality and observability. We would offer our solution as one that best fits in the data mesh landscape to work with your scale, not burden it.